Understanding the Process Behind AI-Generated Images: A Comprehensive Guide
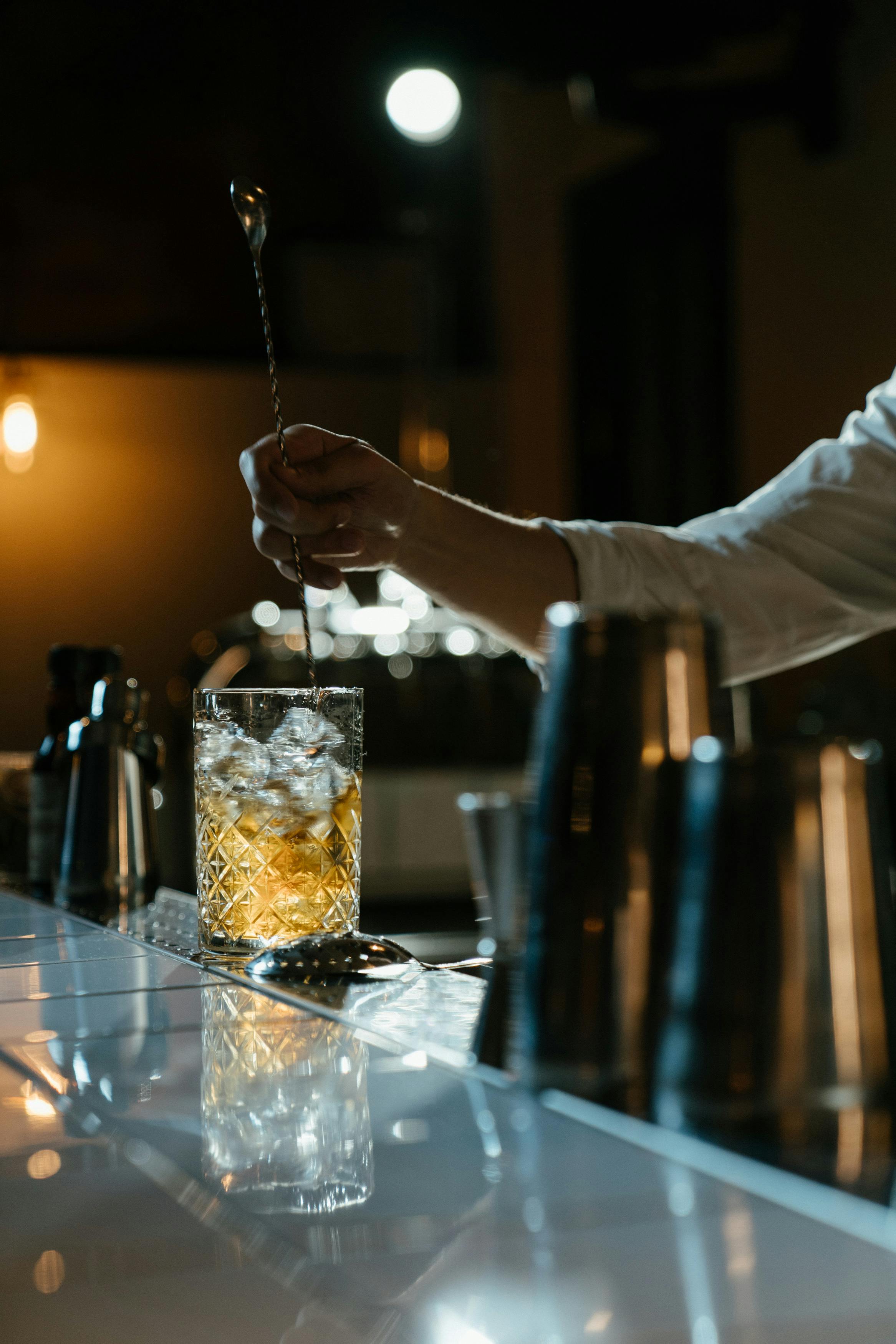
Artificial Intelligence (AI) and Machine Learning (ML) have radically changed our lives, offering innovative solutions from robotics to predictive analysis to automated driving. One of the most exciting applications of AI technology is image generation. Cambridge Dictionary defines generated images as "an image produced by a computer." AI has begun to employ different techniques to create images that are compellingly realistic or abstractly imaginative. To understand how AI manages to produce these images, we will delve into a tour through the workings of Artificial Intelligence in image creation.
Introduction to Generative Models
Generative models lie at the heart of AI image generation. These models are capable of creating new samples from the same distribution as the provided input data. In other words, it's an AI system trained on a vast dataset of images, and then tasked to create similar images by its own. With the help of algorithms and complicated mathematical structures, these models can generate visually coherent images with incredible accuracy. The result is a new instance that matches the patterns and characteristics of the training data but is a completely unique creation.
GAN: Generative Adversarial Networks
Among various types of generative models, Generative Adversarial Networks (GAN) have attracted a lot of attention in the AI image generation field. GANs consist of two components: a generative model and a discriminative model, which work in contrast to generate new data. The generator tries to create images that the discriminator cannot differentiate from the actual data, while the discriminator aims to tell apart the real from the generated. Over iterations, the generator improves its capacity to create believable images.
Neural Style Transfer
Neural Style Transfer (NST) is another exciting development in AI image creation. This method originated from the concept that the style and content of an image could be separated and then manipulated independently. A famous example of NST is probably the algorithm's ability to apply the artistic style of famous painters onto a regular image, giving them a unique and artistic view. NST uses convolution neural networks (CNN) that focuses on picking up textures, colors, and more artistic facets of an image and applies them onto another.
The Magic of DeepDream
Another powerful tool in AI image creation is Google's DeepDream. Instead of generating entirely new images, it retrieves and emphasizes patterns in existing images, creating dream-like, psychedelic patterns. Through repeated pattern enhancement and layer manipulation, the AI system manipulates images to manifest new forms, features, and imagination-defying scenes.
The Revolution of Image Synthesis
The arrival of GANs and similar models have revolutionized the field of image synthesis. From creating realistic human faces that do not exist, to transforming sketches into photorealistic images, GANs have provided fantastic insights and use-cases in several areas, including in art, medicine, and entertainment. Researchers continue to work on improving these models, paving the way for a future where AI can generate images with incredible precision and creativity.
Security Concerns and Ethical Implications
While AI image generation technology presents thrilling potential, it also raises important ethical and security concerns. Deepfakes, a term derived from "Deep Learning" and "fake", uses the AI techniques discussed to fabricate convincing phony videos or images. This technology poses significant threats, such as disinformation spread, blackmail, and identity theft. Thus, it is crucial to implement stringent guidelines and regulations to prevent the misuse of these technologies.
To conclude, artificial intelligence continues to evolve, perpetually pushing the boundaries of what is possible in image creation. The journey of AI in this arena has just begun, and we are yet to witness many more revolutionary technologies in the future. However, the responsible use of these technologies is equally important to ensure a secure and ethical real-world application.