Understanding Hallucination in AI: An In-depth Analysis
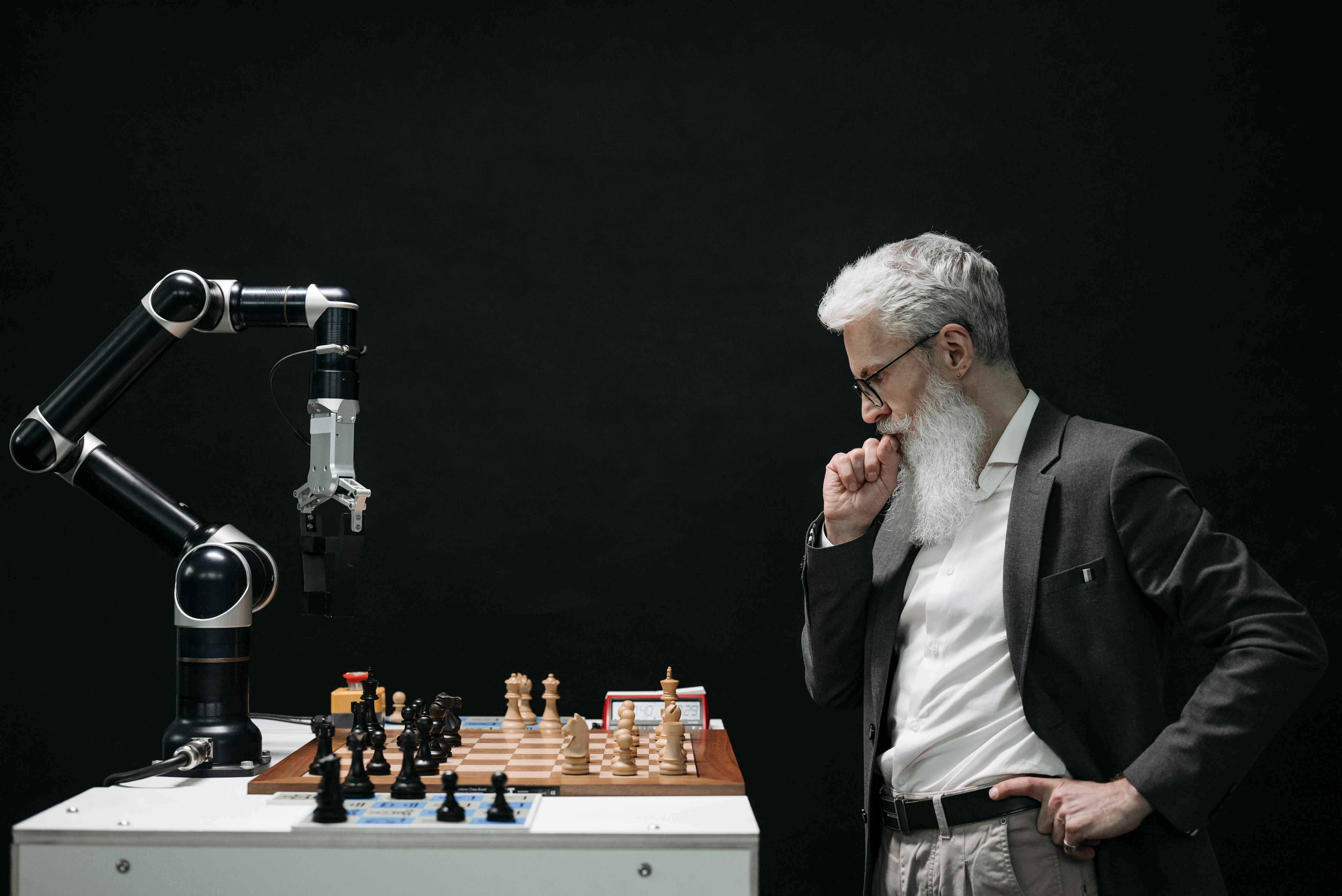
Over the past few years, Artificial Intelligence (AI) has emerged as a key driving force across various technology-based sectors. With its ability to mimic human capabilities like learning, analyzing, problem-solving, and perception in an advanced and sophisticated way, AI holds an impressive potential. This potential is extended to an intriguing phenomenon known as "Hallucinating in AI". The term is being increasingly used in the Artificial Intelligence industry yet remains largely obscure for many. This concept doesn't imply AI systems seeing colorful visuals or having imaginary friends, rather it refers to a specific set of functions and events in AI operations.
Unraveling the Enigma of ‘Hallucination in AI’
The term ‘Hallucination’ in the AI domain is not connected to perceptual distortions experienced by humans, where the brain perceives non-existent entities. Instead, it is associated with a technical phenomenon where an AI system generates an output based on imagined or non-existent inputs. It's an instance where AI predictive models incorrectly interpret data patterns and produce false outputs. These outputs often have no real value or core basis, and sometimes don't even correlate with the existing data, hence the term 'hallucination.'
The Underlying Mechanics of Hallucinations in AI
Hallucinations in AI occur mainly due to the misinterpretation of data during training or testing. When an AI model is trained using vast amounts of datasets, it attempts to learn patterns and correlations. Its main goal is to establish predictive models based on the data it has been trained on. However, sometimes, the AI model might become overly reliant on certain patterns or features, becoming overfitted and failing to generalize well. This can result in the AI model 'hallucinating' data or features that are not truly representative or don't actually exist in the test dataset.
The Impact of AI Hallucinations
The major concern with hallucinations in AI models is the inaccurate and unreliable data outputs. These hallucinated data can be misleading and present significant challenges, especially in sectors like healthcare where accuracy is paramount. In predictive healthcare models, such inaccuracies can lead to incorrect diagnoses and potentially harmful treatments. Therefore, detecting and preventing AI hallucinations is essential for ensuring the accuracy and reliability of AI applications.
Addressing the Hallucination Issue in AI
To overcome the hallucination issue in AI, it's essential to focus on robust model training. An AI model needs to be trained on diverse, high-quality datasets that don't encourage overfitting. Applying regularization techniques can also improve the model's generalization ability, reducing the risk of hallucinations. Furthermore, it's critical to continuously monitor and test AI models to detect any instances of hallucination early and rectify them before they can cause any significant harm or distortion in the data output.
Conclusion
While the concept of hallucinations in AI might seem like an academic curiosity or a novel quirk of advanced algorithms, it carries practical implications that can impact AI performance. Improper handling of this issue can lead to misleading results and harm the overall integrity and reliability of the AI systems. Thus, rigorous training, regular monitoring, and robust testing are crucial to effectively manage and minimize hallucinations in AI. As AI continues to grow and evolve, understanding and addressing this phenomenon will only grow in importance.