Exploring ChatGPT: A Deep Dive into Optimizing Language Models for Enhanced Dialogue Generation
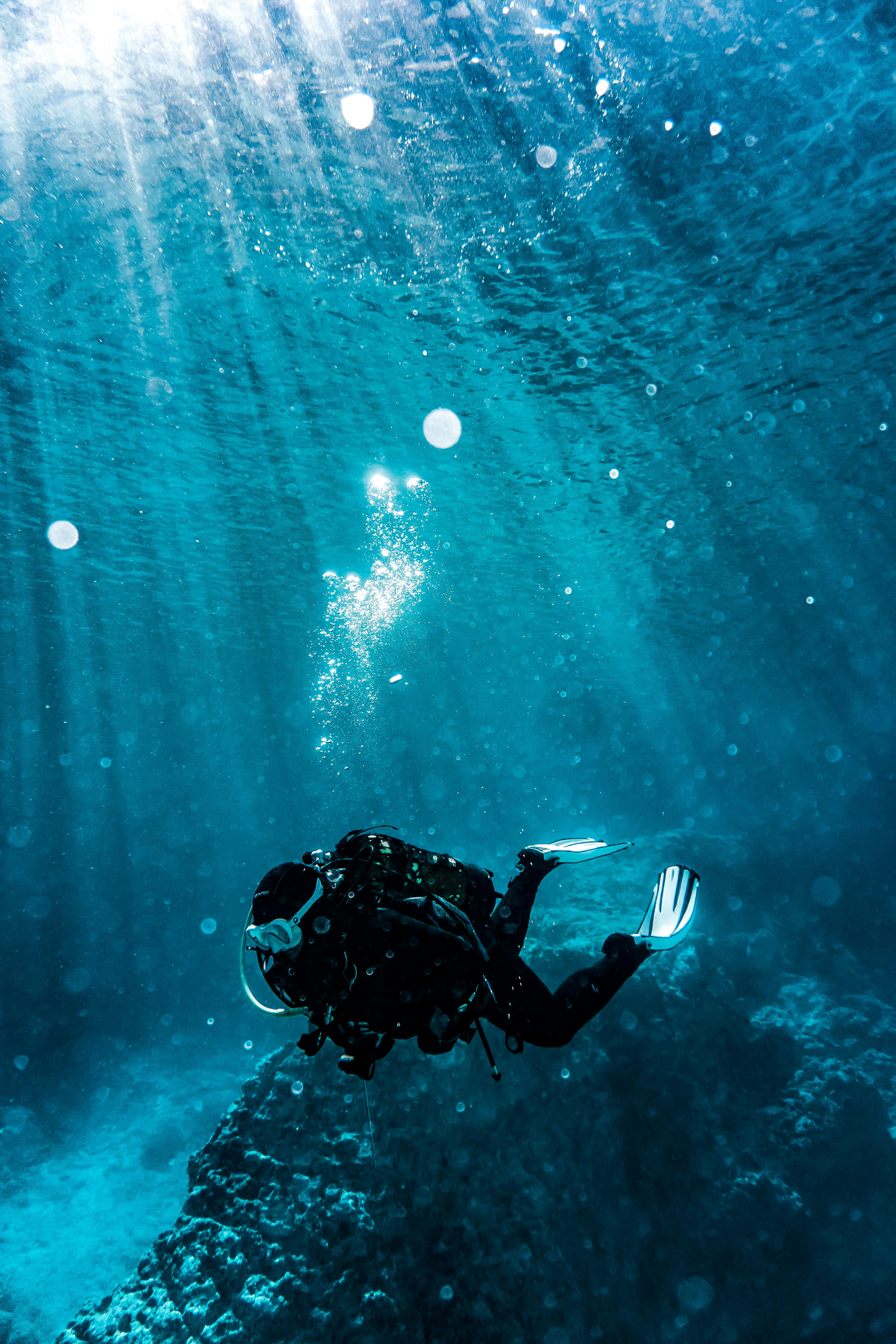
The computational linguistics realm has seen a surge in innovation and progress in recent years, particularly in the aspect of language models. In this context, one of the appealing innovations has been the dialogue optimization in language models. These models are often trained on an extensive assortment of data on the internet, resulting in machines capable of engaging in conversation with human-like precision. This extraordinary transformation unveils a new realm of applications, such as drafting emails, preparing content, tutoring various subjects, translating languages, and even simulating characters for video games.
Building upon a Diverse Sprawl of Data
The training of these language models is often initiated with an extensive variety of data from the internet. However, it is vital to understand that while the model churns out the responses, it doesn't apprehend or access any specific databases or documents. The model's proficiency is purely finessed on the data it has been trained upon, rather than an behest of data search. This predominantly benefits in producing unique interactiveness in the conversational AI, touring beyond mere FAQs or programmed responses.
Privacy and Ethical Guidelines Compliance
In this era of digitization, privacy concerns and ethical guidelines are paramount. While training the models on a broad spectrum of data from the internet, it is inevitable for these models to encounter data or instances that are private, irrelevant or offensive. Strides have been made to ensure user guideline compliance and implement rigorous privacy standards. To this effect, safety mitigation measures have been developed to ensure that the model does not generate inappropriate or harmful content.
Exploring the Pitfalls
While language models adapted for dialogue offer groundbreaking facilities, they also have associated drawbacks. At times, the models may provide information that is incorrect or nonsensical due to inaccurate comprehension. Additionally, they might exhibit biased behavior or inappropriate reactions, a consequence of the model replicating the trends observed while training on internet data. Similarly, while striving to make conversations engaging, these models sometimes overextend by emanating the excessive chattiness, failing to concede when they lack the knowledge about a specific topic.
Creating Safer and More Useful Conversational AIs
Given the authority these language models are poised to have on future interactions, it is essential to design them in an optimized, principled, and user-centric way. This involves addressing their drawbacks and improving upon them. Regular system upgrades, refining default behavior parameters, and enabling user customization are among the key methods of optimizing these models. It's equally crucial to facilitating public inputs on system developments, broadening the perspectives included in system behavior.
In conclusion, dialogue optimized language models herald a new era in computational linguistics. With enhanced privacy and user guideline compliances, and constant overhauling on system behavior, these models are poised to revolutionize artificial intelligence communication systems in the future.